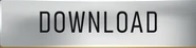
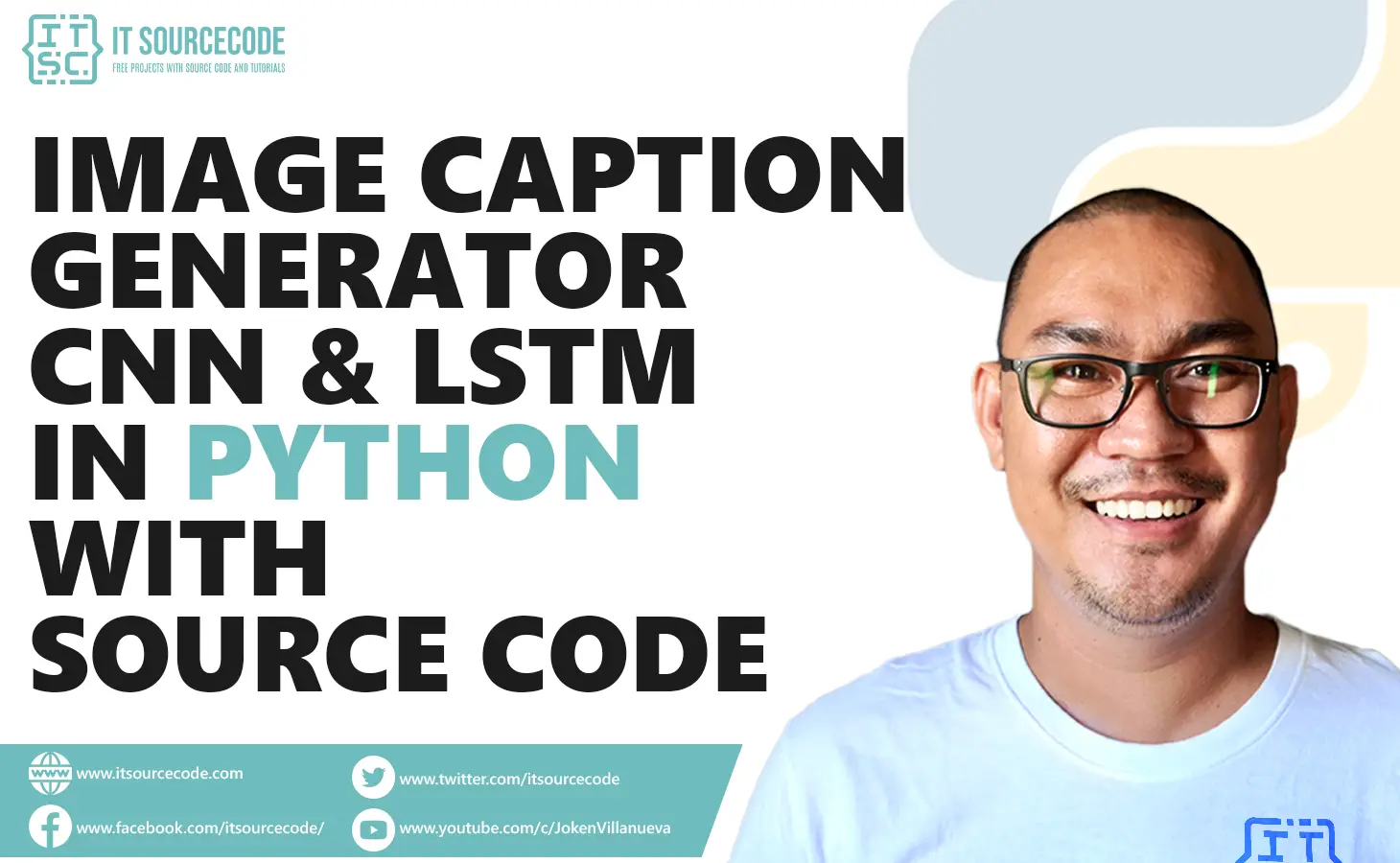
Our dataset consists of posts from Instagram users that follow our accounts: and Due to the fact that the scraper required logging into Instagram via Headless Chrome, we were only able to access the information from users we follow and public profiles. We wrote a simple script to parse each JSON in our scraped profile directories, and from there, we extracted Instagram photos and captions. We scraped Instagram using a profile crawler powered by Selenium that we forked from GitHub user The scraper built JSON objects for each Instagram user, including post information, location information, comments on each post, the timestamp of each user interaction, and number likes, among other things. This blogpost assumes familiarity with basic Deep Learning concepts such as convolutional neural networks (CNNs), recurrent neural networks (RNNs), gradient descent, backpropagation, overfitting, probability, Python syntax and data structures, Keras library, TensorFlow, etc. To achieve this, our model consists of a Convolutional Neural Network (CNN) and a Long Short-Term Memory (LSTM) Recurrent Neural Network (RNN). Our work aims to generate captions that follow a specific style with specific vocabulary and expressions. In this case, user posted the above image with the caption “aarhus goes way 2 crazzyyy”. In some cases, the caption may not be relevant to the presented image at all. Instagram captions tend to be more advanced than a simple descriptor and consist of puns, inside jokes, lyrics, references, sentiment, and sarcasm. Isn’t that how you would describe it as well? But if you wanted to post this image on Instagram, would that be the caption for it? Microsoft’s CaptionBot answered with “I think it’s a group of people posing for a camera”.

CAPTION GENERATOR GENERATOR
A simple caption generator could describe the image as something along the lines of “four friends in a pink room”.
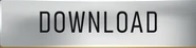